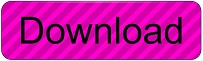
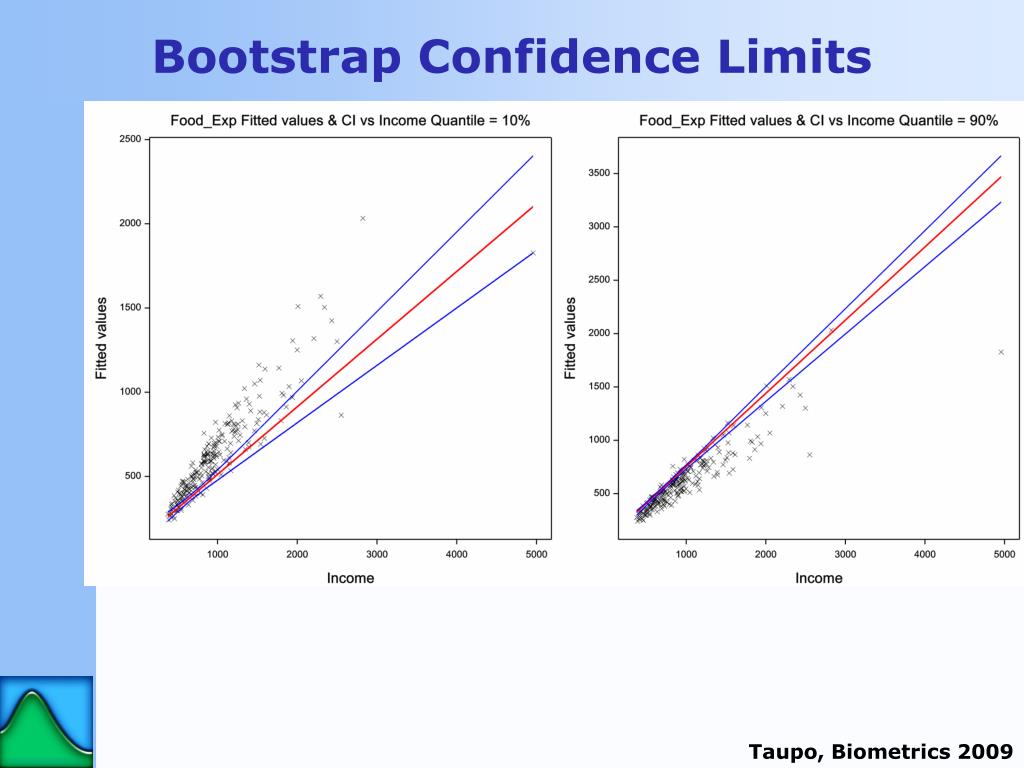
Both theories support that the direction of causality is from X to Y and not from Y to X. The idea is to see how both these variables affect Y along the quantiles of Y and to test both theories. Another suggests that X should have negative impact (becoming more negative for larger values of Y) and that X' should have a positive impact and larger as Y increases. One theory suggests that X and X' should both have a statistically significant and positive impact on Y (dependent variable). X is an input factor in a country-year (hence panel data specification) and X' is another input factor. I expect X to only have an important impact on Y for high values of X (and for another variable, say X',I expect the opposite to hold). I expect that the impact of X on Y varies significantly across the quartiles of X. I am trying to see how X impacts Y for given quartiles of X. What is the difference between the two approaches and in which cases would one be more appropriate than the other?
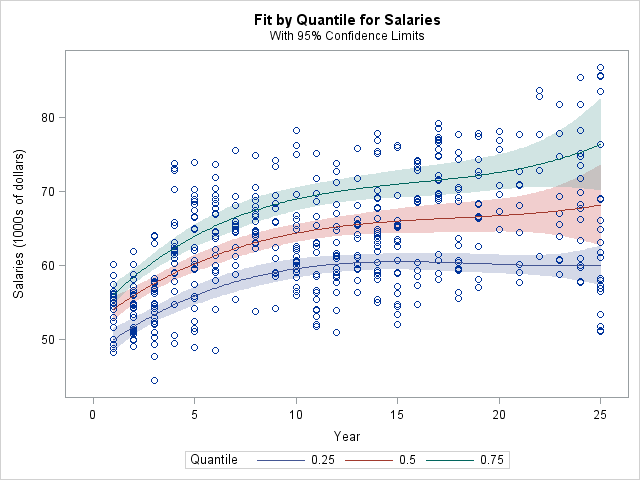
So basically we would have y = intercept + D0.5*X + D0.75*X + D1.0*X + controls, where D0.5 is the indicator variable for the second quartile, D0.75 is the indicator variable for the third quartile, and so on. It is a far-reaching generalization of the median regression, allowing for a predition of any quantile of the distribution. Use OLS regression to regress Y on the quartiles of X by using interaction terms, that is, multiplying X by an indicator variable that takes value 1 if the observation belongs to a certain quartile. Quantile regression, introduced by Koenker and Bassett Jr ( 1978 ), has become a very popular tool for analyzing the response of the whole distribution of a dependent variable to a set of predictors. I want to regress a variable Y on another variable X (with appropriate control variables and fixed effects) in a panel data setting.
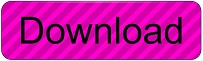